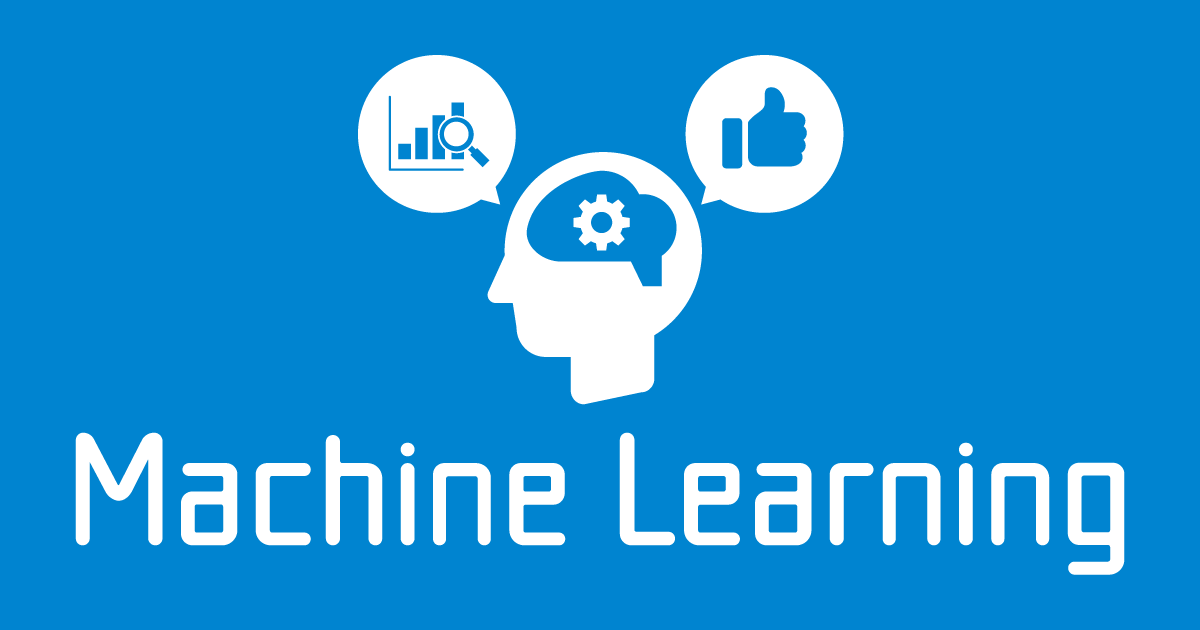
TensorFlowの構造化データセットをnumpy.ndarrayに変換する
皆さん、こんにちは。クルトンです。
今回はTensorFlowのデータセットをnumpy.ndarrayに変換してみました。 簡単に他フレームワークなどの動作確認をする場合に使えるかと思います。
今回行なっている環境はGoogleColabでランタイムはCPUです。
TensorFlowのデータセット準備
まずは必要なモジュールをimportします。
import tensorflow_datasets as tfds
次に、データセットを読み込みます。使えるデータセットは公式がまとめているのでこちらをご確認ください。構造化データセットはStructuredと書かれているところのものです。色々なものがあります。
例として、今回選んだデータセットはペンギンについて調べたものになります。 詳しくはこちらをご覧ください。
実際にデータを読み込んでいきます。
tf_dataset, info = tfds.load("penguins", as_supervised=True, with_info=True)
ここでデータの本体はtf_datasetという変数に格納されています。データの概要をコード上で確認するため、infoという変数を用意しています。
infoをprintメソッドを使ってデータの特徴を確認するとこのようになります。
tfds.core.DatasetInfo( name='penguins', full_name='penguins/processed/1.0.0', description=""" Measurements for three penguin species observed in the Palmer Archipelago, Antarctica. These data were collected from 2007 - 2009 by Dr. Kristen Gorman with the [Palmer Station Long Term Ecological Research Program](https://pal.lternet.edu/), part of the [US Long Term Ecological Research Network](https://lternet.edu/). The data were originally imported from the [Environmental Data Initiative](https://environmentaldatainitiative.org/) (EDI) Data Portal, and are available for use by CC0 license ("No Rights Reserved") in accordance with the Palmer Station Data Policy. This copy was imported from [Allison Horst's GitHub repository](https://allisonhorst.github.io/palmerpenguins/articles/intro.html). """, config_description=""" `penguins/processed` is a drop-in replacement for the `iris` dataset. It contains 4 normalised numerical features presented as a single tensor, no missing values and the class label (species) is presented as an integer (n = 334). """, homepage='https://allisonhorst.github.io/palmerpenguins/', data_path='~/tensorflow_datasets/penguins/processed/1.0.0', file_format=tfrecord, download_size=25.05 KiB, dataset_size=17.61 KiB, features=FeaturesDict({ 'features': Tensor(shape=(4,), dtype=tf.float32), 'species': ClassLabel(shape=(), dtype=tf.int64, num_classes=3), }), supervised_keys=('features', 'species'), disable_shuffling=False, splits={ 'train': <SplitInfo num_examples=334, num_shards=1>, }, citation="""@Manual{, title = {palmerpenguins: Palmer Archipelago (Antarctica) penguin data}, author = {Allison Marie Horst and Alison Presmanes Hill and Kristen B Gorman}, year = {2020}, note = {R package version 0.1.0}, doi = {10.5281/zenodo.3960218}, url = {https://allisonhorst.github.io/palmerpenguins/}, }""", )
features=FeaturesDict({
と書かれている部分の内容を確認するとどうやら、features
というところにペンギンの特徴がfloat型で記されており、species
でペンギンの種類が違うものをint型でラベルづけしているようです。
tf_datasetのキーを確認
まずはデータにどのようにアクセスすると良いかを調べます。
tf_dataset.keys()
上記コードを実行すると、dict_keys(['train'])
という結果が返ってきます。どうやらtrain
というキーを入れるとデータが取得できそうです。
ただし、PyTorchなど他フレームワークで使うには、データセットの型を変換する必要があります。
データセットの型をnumpy.ndarray型へ変換
numpy型に変換
dataset=tfds.as_numpy(tf_dataset['train'])
これで、dataset変数にnumpy型でデータが格納されました。しかし、さらに変換が必要です。 dataset変数には、データとラベルが一緒に格納されているからです。
dataとlabelで保持する変数を分ける
feature_data, label_data= np.array([x for x,y in dataset]), np.array([y for x,y in dataset])
これで、feature_data変数には2次元配列でペンギンたちのデータが、label_dataには1次元配列でペンギンたちの種類が格納されました。
ここまでの変換作業をする事で、他フレームワーク(PyTorchなど)でも使える状態まで持って来られました。 あとはモデルで使うのに必要な通常行うデータの処理をすれば実際に使用できます。
今回は一例としてモデルの学習を行い、その後の予測まで行います。具体的には、このペンギンのデータセットを使ってLightGBMを動かしてみます。
LightGBMで動かす
モジュールをimport
学習させるまでに必要なモジュールを先にimportします。
import optuna.integration.lightgbm as lgb from sklearn.model_selection import train_test_split
データセットを3つに分ける
用意したfeature_dataとlabel_dataを使って、学習用データ、検証用データ、テスト用データの3種類に分けます。
x_train, x_test, y_train, y_test = train_test_split(feature_data, label_data, test_size=0.2) x_train, x_val, y_train, y_val = train_test_split(x_train, y_train, test_size=0.2)
モデルの学習
LightGBMで渡すように学習用と検証用のデータセットをlightgbm.basic.Datasetに型変換します。
lgb_train = lgb.Dataset(x_train, y_train) lgb_val = lgb.Dataset(x_val, y_val, reference=lgb_train)
今回は3つのクラスがあるので多クラス分類で学習するようにパラメータを設定します。その後、データセットと設定したパラメータを使って学習します。
params = { 'objective': 'multiclass', 'num_class': 3, 'metric': 'multi_logloss', } lgb_model = lgb.train(params, lgb_train, valid_sets=lgb_val, )
予測の結果を出力
学習したモデルを使ってaccuracyを出してみます。
y_pred=lgb_model.predict(x_test,num_iteration=lgb_model.best_iteration) accuracy = sum(y_test==np.argmax(y_pred,axis=1))/len(y_test) print('accuracy: ', accuracy)
結果はaccuracy: 0.9552238805970149
となりました。
おまけ
データセット検索サイトをまとめています。今回使用しているようなデータセット以外で必要なデータセットがあれば、以下のようなサイトで検索してみると見つかる可能性があります。
終わりに
今回はTensorFlowのデータセットを使って、LightGBMモデルを学習させるところまでやってみました。
scikit-learnやPytorchなどにもデータセットがあります。今回はそれ以外で色々なデータセットを使ってみたいと考え、さまざまなデータセットを取り扱っているTensorFlowを使ってみました。
今回は使わなかったのですが、Amazonの商品レビューのデータセットなどもあり、モデルの性能確認をする時に複数のデータセットを用意するのに使えそうです。
今回はここまで。
それでは、また!